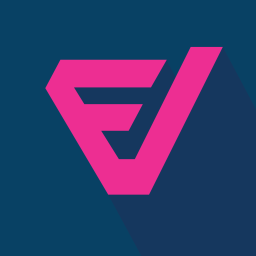
Guillermo Jose - AI Ethics
Founder's Voyage ·
00:00:00
00:00:00
Transcript
00:00:00
For me, AI is like a knife. Is a knife good? Well, it depends how you use it. If you're going to kill someone, it's bad. But if you are going to prepare sushi, maybe it's good.
00:00:26
Our featured speaker today is a data scientist and software engineer with extensive experience in the healthcare industry, an MIT bootcamp alumni with a solid grounding in AI and machine learning techniques and passionate about leveraging his skills and passion to create AI solutions with global application across industries. Guillermo, it's a pleasure to finally have you on as our featured guest today. Thank you so much for taking time to share your journey with us. Thank
00:00:59
you,
00:00:59
Nancy. So I know that we know each other to a degree, but I was wondering if you might just lead us off talking about some of the earlier experiences in your life or some of the people that came into your life early on that you really feel had a strong influence on who you've become.
00:01:20
Well, I think that the first person that had a big influence in my life early in my life is, and I'm going to be very short on this, was my father. He was a scientist, but also an applied scientist. So he was a chemical engineer, but also a person that was able to apply science to build businesses. I grew seeing him applying science and building businesses. So that's where my first insight and understanding of how to science or mathematics or whatever can be applied into the real world. That was my first example. And then during the years, whenever, every place that I go, I have someone that teaches me something. It doesn't matter. It can be like any person, like people that I would never expect. The that I do is I try to always be humble and try to listen and try to learn from everyone. From consulting, I learned from the designers, right? that I didn't know that they were going to teach me something, but designing the slides at that time was very important. So okay, these are the colors, this has to be like this, you because this is not a line. And I was like, looking at the deck and why, why is not the line? I don't see any misaligned.
00:02:37
When I was at Deloitte, I learned very much how to pay attention to detail to those small things. Later, that will help me paying attention to detail when I was doing graphs, being a data scientist. Then, one of the most important persons that had an influence in me was a professor when I was at Carnegie Mellon. His name is Mike Smith. I took several classes with him, with one about digital transformation. So showed me that, or he taught me that technology was not everything, and that you have to pay attention to so many more things in order for that technology to be really successful. So I work with him in several things. I with him doing papers, also doing research. And I learned very much about paying attention not only to the technical things, but also to the human element, to the context and everything. So he was a big influence later in my life, right? I don't know those, I think the ones that I remember.
00:03:33
My last boss has also been a big influence in my life too. So everywhere I go, I had an influence.
00:03:41
That's amazing. I love that, um, just mindset, right? Of everyone can teach me something and I want to learn from everybody. So how can I stay humble and listen and interact with people in a way that I have something to learn from them just as much as they can learn from me. So it sounds like you've been doing that throughout kind of your career and your, your life for that matter. And it's kind of helped you along the way. And it sounds like from what you've described and a little bit from, we talked about this as you kind of have a path that's a bit varied, right? So, you know, you talked about some people analytics. You talked about software engineering, some design. So really interested in how you decided to get into software engineering, data science and AI. Why was that the field that you gravitated towards?
00:04:21
Well, you always make mistakes in life, right? So my first mistake was like asking people when I was like 18 or 17 years old, like ask advice to people that I shouldn't and people that they didn't know about how life really worked because of that. And I don't want blame but guided by something so I began choosing to study accounting. So then that was my first mistake. You
00:04:47
know what I've gotten that same advice as well so you're not the only one.
00:04:50
So I went to finish accounting but then I had this like little idea and I saw my father how he has built businesses around science and it was funny because when I was studying accounting I would take my physics books or my philosophy books and I didn't care about the classes. I was always reading philosophy or mathematics. And that was very helpful because it was like, it gave me time to study everything that I wanted, right? While I just needed to pass an exam at the end of the semester, right? Like about something with accounting. So I remember very well reading this Mr. Tompkins behind the atom and things like that, that really sparked my imagination. But then after that, I went to study mathematics and philosophy and mathematics, and then I changed my path again.
00:05:39
But it was very difficult, as I didn't have... I had to begin from the ground up, like from the base. That is how I began understanding that one of the most, what the opportunities were in the intersection or in the interaction of several kinds of knowledge, let's say like that. When you mix, for example, design and mathematics and philosophy, then you can have a breakthrough, right? Like how you can see and how you can connect those dots. So for example, when I was at CMU, one of the things that I did is I research to see if there were a logic of creativity. If there's a logic of creativity, then you would be able to symbolize that and to use that logic to create tools that will foster the new product development, creativity, innovation. And that will also allow certain societies to close the gap between differences. So that was one of the things that I realized since I was very young. And being able to study so different things like design and accounting and then mathematics and then philosophy, that was the thing that allowed me to see how everything works together. So when I was doing consulting, I could see how you could use natural language processing to review certain things in accounting and to be able to audit and make the process of auditing faster and better, right? Because science also can be used to do that. So everything is useful.
00:07:15
That's wonderful, and I love that you're at a point
00:07:17
where you can see how it's all kind of interconnected and shaped your journey. So how did this lead you into AI? And like what aspect of AI kind of fascinated you first?
00:07:30
Well, the grounds of AI is first of all, is built in ideas. And the first ideas were these ideas of teaching a machine how to think, or how can I make this thing how to think? So the first approach to AI was to use symbolic logic or this symbolic approach to train machines to think. One of the first places that this thing, AI, was created was at Carnegie Mellon. That's one of the first places that the field of artificial intelligence, and it's still up today, I think that CMU is good on that. So there was these guys that's called Simon and Ewell that invented the first AI, like tools and robots, and the first approach was to use rules and symbolic logic to teach a machine, for example, how to play chess. When you understand the logic and the philosophy behind it and then how you can link, because at some point philosophy and mathematics are the same. I don't want to get into very technical things, but this was demonstrated by a guy that's called, his name was Korketel. He did for mathematics what Einstein did for physics, right? so he demonstrated that any system as big as arithmetic, it's inconsistent always. You can even either achieve completeness or consistency. And that's what makes me think that AI is never going to take the human being or take everything that a human being can do, right? But that doesn't mean that it's not going to display some of our work.
00:09:06
But going after that, going back to your question, that is being able to join the points from Connect the Dots, from philosophy, mathematics, and how to apply, how to solve a problem. That's what brought me interested first in computer science and then AI. Why AI? Because I'm very lazy also. I have to do things that I don't like doing. right? Like when I was doing administrative reports or things like that. So I'm always trying to, if I can automate something, I'll do it. That happens when I was working at Highmark, and I hope nobody from Highmark listens to this, but when I was working there, I was taking maybe a week doing some things and doing some reports and something like that. So I said no. I took a process and then it's okay, two hours and then my boss will come and how much, like maybe tomorrow, so I had time also to develop other things at my work. You know, it's better to work smart. So that, that's what also brought me to AI, but also the possibilities of making this world a better world. Right? So the potential that has AI to close the gap and to make this a better world. For me, AI is like a knife is a knife booth. Well, it depends how you use it. If you're going to kill someone, it's bad. But if you are going
00:10:35
to prepare sushi, maybe it's good. That's what also brought me to AI because I can see how AI can help very poor communities or poor people around the world. That's the other part that brought me to AI. And that's what I was telling Matt, that connection of that, how to use AI, because I think that AI will potentiate like really the part of behavioral analytics. And that behavioral analytics is what many people like many underserved communities in the world need.
00:11:11
I I love that just kind of your stance on AI and how you, you know, you want to apply it. I certainly wholeheartedly agree with your and align with your, I like to automate things because I'm inherently lazy. So how do I make my job easier and then put some buffer time to work on projects that I want to work on? So I love that that's kind of where it started, right? Kicking that off, it was really that idea of like, how are we more effective, more efficient? And in so many words, it's how do we help humans, right? How do we help people be better, whether it's you being better or your company being better at the work they're doing, help underserved communities through AI. And so I love that that's your reason why you gravitate towards it. I think it's extremely altruistic and can certainly see some really positive application of AI in that. I do want to ask a little bit about, on that AI thread, one area that the community's talked about. We've had several topical discussions on AI itself. Obviously, you mentioned this a little bit, is it's how you use it, right? And so there's some ethics around it. Do you have any thoughts on ethics of AI or how AI should be used? Where do you foresee AI going as we steamroll towards this? I mean, I think we're in a really interesting place right now with AI where it's kind of the same place we were at probably 10 years ago with data science, right? Everybody thought they wanted to do it. Everybody was trying to do it. Nobody really knew if they were doing it or not, but everybody wanted to put it somewhere, right? So we'd love
00:12:32
to get your thoughts on just AI in general.
00:12:35
That's a huge topic. I'm going to be very direct on that. Do I think it needs to be regulated? Absolutely. The second thing is I think there's responsibility in the people that are building those systems. Because if you embed into the models those biases, that's going to perpetuate that bias. And I'm going to give an example. Let's say that you are trying or you go and ask for a credit into a bank and then it's in the regulation written that you cannot use race to predict credit worthiness. But then if you use the zip code instead of the race to predict those credit worthiness, as it's a confounding variable and those are correlated, you are basically using race, right? Because there, if you, for example, if you use the zip codes in Boston, maybe that are in Dorchester, right?
00:13:32
you are going to predict race in some way. So those are biases that are embedded into the model. So we need to be very careful and be very aware of the things that we are embedding into the models to be able to see, okay, is this bias or not? Because the machine doesn't understand anything about biases. It's about how you feed the algorithms. There are some algorithms that will perpetuate that bias more than other ones. But it also has to do with how the data scientists train those models. So, there's a human responsibility behind that, always. So, we cannot say, no, it was just a model that learned about it. No, there's always a human or somebody that makes that decision for that to be trained in that way, right? So, yes, I absolutely it has to be regulated. Yes, absolutely. It has to be controlled in some way because also it's a tool. There are two or three types of AI that are now there. There's a difference between machine learning and large language models and foundational models. The difference is that with machine learning, we have control, like direct control to it. But with large language models, as you need to train billions and billions of parameters and huge amounts of data, there are only a few companies that could do that. So if they achieve those foundational models, like chat GPT or cloud or whatever, they have the power, right? So they control that technology and controlling that technology comes with a lot of responsibility, right? And we saw the news, we saw what happened with Altman. And I'm sure he was doing things
00:15:18
that were not the most transparent, but I'm not going to get into that politics. The ones that control that would control many things and would have the power. That's why Google also has been so interested in Microsoft not losing that power. Those are like Game of Thrones with AI. There has to be some open source, but also balance that power. That's also another part of the AI ethics. Right? So you have the microcosmos in which a data scientist that is training a machine learning model has to be responsible for the biases that it can embed. But also, we have to contrast the power on the other side, on the large language models,
00:16:03
those foundational models, how we control and balance that power and that ethics. But yes, of course, human beings, and of course, we have complete responsibility of what we're doing because you cannot say, oh, no, they're like mathematicians that say, let LLM be. No, if there's someone created that thing, it's not like out of the blue, you know? So there's always some responsibility behind it. And we are responsible for that.
00:16:29
I your answer. I honestly think you're going to have to write an answer for a PR firm someday because it's a difficult topic, you know, to say, this is definitively the responsibility of the creator, this is definitively the responsibility of, you know, this governing body to regulate because I can see as AI evolves, that will probably evolve along with it.
00:16:56
And embed, but also we have the control of power that on the other side, on the large
00:17:03
language models, those foundational models, how we control and balance that power and that ethics. But yes, of course, human beings, and of course, we have complete responsibility of what we're doing because you cannot say, oh, no, they're like mathematicians.
00:17:22
I really appreciate your thoughts based on your experience and your passion for this subject. And given that you have so much experience and passion about AI and taking your understanding of data science. What is the problem that you would seek to solve using AI if you were to, and is there like a sort of business model that would interest you to create to solve that problem?
00:17:50
As I said, that's a very difficult question, and it's because it's not that AI is just a business model. It's like AI can be parts of a business model, it can enhance a business model. But for example, I can see how AI can be used in healthcare for medical adherence. Like for example, for people, I've been seeing and reading cases about how AI was able to save lives, right? For people that were misdiagnosed so that they couldn't be diagnosed in the correct way, as large -angle models were trained in that specific cases, they would be able to link the knowledge and the dots of a huge universe that the human being could not do. Again, following Herbert Simon and the founders of AI, there's something that's called bounded rationality. That means that your rationality and your capability to process information has certain limits. There was this guy that had, like, all the symptoms of a heart attack, right? And he was almost having a heart attack, and he was diagnosed by one doctor, and then by other doctor, and they say, okay, you need to have a procedure. But they couldn't find what was happening, and then they asked the AI, and they say that there were small cases that would have a congenital thing here in the throat or something like that. And at the end, it was that. And it was like, okay, if it's not all of this, there has been a small cases that has been proven to be this. And because of that, they realized that that was what he has. Some doctors didn't even know that that could happen. And that AI using it as a tool in the path or aid to the patient was able to save his life. That's just one example, but I can see many applications more. Right? I can see, for example, for underserved communities, like how to give credit to like this being certain models that have been applied in many different parts of the world, like India and Pakistan, like how to give credits to women and create communities, right?
00:19:56
In which it creates virtual loops, but then the AI can help you measuring from every point in time how you are doing, it can give you advice, it can make you more success because it can see things that you can't. For example, when you were talking about a business owner, it's not going to substitute all your business tools, but it can be like the links between certain processes in which, okay, we're going to do this, but then we're going to give the ball to the AI to do this, And then we're going to receive the output, process it again, and then give it again to the AI. So those are something that they'll call agents. So agents are very well -trained nodes of AI that can do very well a task. Like for example, you were saying, I don't have time to read my email. It's too much.
00:20:49
I have thousands of emails. You can create an AI, an agent that will read your emails and based on your priorities will tell you every five hours, okay, summary of your emails from this time to this time is this, your priorities are this, based on what I know from you, this is this and this, I recommend you this. So then it's an aid and it's something that leverage or that can leverage, you can use as leverage for your work and not necessarily substitute you in the whole process. But if it can give you two or three hours of your day, every day, it's a huge win. It can be applied to, I don't know, like the use cases are, for me are huge. Like it's so diverse, the important thing is to not only being, or not to focus so much in like being an expert in the programming part, but also to be able to see the whole picture and see, okay, here it fits, here it fits, let's create, let's build that solution.
00:21:47
That's how I see it. That's great. I the thought there, and really it's just augmentation to our current work to make us more efficient, it sounds like, and help us, I don't want to say make the right choices, but if we see error in process, for example, it can help us course correct, right? And I like the way you phrase that as agents, right? And so being able to just get time back to do things that are more valuable. And was, and I'm going to blink on the name, but there was a gentleman who was basically saying just that, is that we need to be spending more time on things that matter, that are important, that are driving the needle forward and what we want to do to the point where he just doesn't answer emails except for one period of time in
00:22:26
a week. And if he doesn't answer yours, sorry. And so that's, to your point, a great example of how AI can help facilitate that. And I think his stance on AI was that if you are currently in a role where you're moving information from point A to point B, and that is your sole job, that AI can take over your job, which I thought was really interesting. But one thing I do want to ask you a bit about just AI in itself and kind of some predictive modeling that we'd be thinking about is the understanding of it, right? So in a social context, people hear AI and they think of generative AI first and foremost, I'd assume, right? Chat GPT, what they've heard in the news. So what challenges or roadblocks have you seen or you'd encountered with people who you're working with or you're working through in terms of not really understanding data science, not really understanding what it is, but thinking they know about it. And then what specifics do you wish that they would understand? What are those few points or tenets of AI that you wish people would just kind of understand of what the foundation of it is to help kind of gain more knowledge around it?
00:23:29
The is that I'm seeing with data scientists and people in the field, the same problem that arose when you were using a calculator. What happened? You maybe understand the procedure of how to make a division. And then you can use a calculator to
00:23:45
four divided by two, right? And you will have to, but then I've seen people that don't understand even in data science, the difference between a rate and a ratio, for example, right? So I've been in interviews in which I ask the interviewee like, okay, tell me they can do it like I call them dot feed predict data scientists, right? Because they can take some code from Stack Overflow from JGPT, but they are not really understanding what they're doing. So when I ask them where those are, like the equation from a linear regression comes from, they don't know. So on misunderstanding the fundamentals and not knowing how things work could be very dangerous. You can run a regression model with Python, but really don't know what you are doing when you are tuning, for example, the parameters or how gradient descent works or things like that. The thing that we are using or that we are having those tools, you cannot let that substitute your brain. And it's like the same case with drivers using Waze or Google Maps. They don't even know the streets anymore. They need the aid of that, so no, they cannot arrive to that place.
00:25:02
It's the same. You need to know the fundamentals and the principles of what you are using. Yes, it's faster, it's easier in some way, but you need to understand what's behind it and the logic, because if not, you can apply something wrong and then you can cause a lot of problems. That's one of the big problems that I see. And the same happens with large language models. if you really don't understand how this thing really works, you are going to think that it's intelligent and that it's really having awareness and context, when it is not. It's like large language models today and based on the architecture or the transformers architecture that they're using is deep learning, and they're using a probabilistic approach. There's no symbolic, there's no connectionist approach, so they are not really thinking. They are very good at predicting. So what you are saying is an output of probability well -applied or applied well, and that's it. It's super powerful. I'm not saying no, but you need to understand and see
00:26:06
what you have in front of you. If not, you are making a God out of nothing. Again, those are the problems that I see in data science.
00:26:14
I really like how you articulated that too, because I feel like that is a way in which it could be explained to the average Joe or Mary, such as myself, because I think that maybe there's been an inherent assumption for a long time that large language models are inherently intelligent and have some intelligence that the rest of us, you know, are going to succumb to. I think that that's where some of the fear comes from that and giving something a robot face. And I know that you and I have talked a lot about how there is fear of AI, like the big question, like, is it going to take our jobs? And that question gets floated a lot in a lot of different respects. If you were to kind of picture like an ideal evolution, how do you envision that like in
00:27:12
positive way AI could transform the way we work or people think about work?
00:27:18
Well, I think if you see it as an aid, right, like as a complement to your work, that's super powerful. And I'm going to tell you an example, specifically in my work. We used to have five people, like external in other country that were helping me to do like some statistical analysis or exploratory data analysis, right? But then I learned how to use chat GPT well. So now my productivity in my pipelines, being a data scientist using chat GPT is like 10 times faster. So what's happening there is not like,
00:27:58
chat GPT is not like taking the job of anyone. It's just making me more productive and making me more productive. that means that I need less. And that's what's going to happen. It's going to augment your capabilities. But the problem with that is, in order for you to use ChattyPT well or any of these AIs, you need to know the field because it makes mistakes. And then you can tell, for example, two days ago, I was doing an analysis and I wanted a very simple analysis, trying to substitute or to reduce our categories in a column into a new column because the categories in one column were less like 60 and I want it to be reduced to 20 so then I can convert those to dummies and be able to make a better model with like regression, right? I told the AI, I need to substitute this and these are the categories. And it was taking a dictionary that was this huge and the code looked horrible. I said, no, no, no, no, no, no. Use the lambda function, do it like that. Okay, now I understand. And then the code reduced to something like this. But you need to know that there exists a lambda function that can do that mapping. Sorry, but maybe very... I changed the way in which the mapping was done. If I didn't tell the AI, the AI would do what it wants. Now that I did prompt engineering and I told it how to do it, it was faster and better. But I had to be aware that there was that possibility. If you are not aware, if you don't know what you don't know, that is like, okay, there's no way I can handle this tool.
00:29:39
That's one example.
00:29:40
You up a really good and really interesting point there, just with that example, because I've run into the same thing, right? Just as, as I've been, you know, I'll have it check code for me or, or, you know, or to your point, if I need to do something quick, like find variables and replace. Yeah, I can do that. But, um, you do bring up a really good point there of a couple of things. One is, uh, knowing the underlying principles and the underlying, um, sources that it's using, because in theory, it's just pulling from its, you know, knowledge base that it's gathered and been trained on and giving you what it knows, yes, I've got two questions a little bit. One is, are we in a point where you still get the same answer, right? So to your example is, is yes, the way you had it is probably much more efficient, more effective, takes less time. And I agree that you need the underlying fundamentals, but if you're getting the same answer the other way, that's longer, is that changing really the. The output of it is that fundamentally changing
00:30:35
the, it It depends because there are certain algorithms, like if we are going to go to algorithms, depending on which kind of algorithm you're using. You have something that's called big O notation. And when you are beginning and you are doing something simple, it doesn't matter. But when you are doing or handling big data sets, using an algorithm that has a linear growth versus one that has an exponential, it will make a difference. So you need to know and tell this guy, use a linear one, don't use the other one because it's going to take two weeks to do this, you know? No, no, no.
00:31:14
That's kind of what I wanted to get at is a little bit of that functionality and thinking through what are the actual causes of it. So I love that answer because it's absolutely true. The other question kind of on that note is, and you mentioned this a little bit, is is a tool to make us faster, right? Obviously, it's helped you. You said you had a team of five that was helping you do EDA and kind of think through your, your data analysis. And that's kind of obviously since been lessened, I guess, how do you see this as this makes us more efficient in some ways, it also, I guess, creates this. Environment within a job or a role that we're expected to be doing more and more and more. Where do you find that balance? Like, how does someone find that balance of yes, I can use these tools, but I only can work, I can only do so much regardless of how efficient I can be, you know what I mean? You hit a point of diminishing returns a little bit there. And so like, how do you find that balance? So one, where do you think that's headed towards? And two, I think in your life, you know, as you think about being more effective and more efficient with AI, how do you keep that balance where you're not constantly trying to do more faster, and maybe I think do more faster and then not being able to get as much done? Is that kind track? I
00:32:29
think the first question, it depends on the nature of work. If you're going to be
00:32:34
cleaning data all day long, you don't want to do that. So it's like the data grangling, you can leave it to the AI. It's horrible. That's why they say that 80 % of our time is spent, like we spend that data grangling. But those kinds of repetitive tasks, I think that AI will be very But then there's a point in which you arrive to making decisions that are based on ethics or that has to do with design or that has to do with seeing the whole thing. You still need the interaction and you still like when you're doing software engineering, I don't think the AI is going to be able to replace a team of software engineers because you need more than just programming. programming, you need more understanding the interaction with a client, the UI, the UX, how people feel. It's not only about programming. You can see that when you read the job descriptions of people that are doing software engineering, the more senior you are, the more you have to manage or handle complex system that there are not only programming too, right? And on the other side, how I feel it, at least with myself, for me is like pasta. If I eat more pasta every day, I feel guilty. So the same if I use AI and I know I'm using and I'm cheating myself, I feel stupid. So I need to go back and say, no, I need a little bit of thinking, right? Like at least maybe doing some math or something. There are some, I don't know, like it was some Russian leaders and also I think Abraham Lincoln or someone like that.
00:34:11
Like, he always in his trips brought a notebook and he was doing geometry and mathematics because that sharpened his mind. It's not only me. I work with people at McKinsey and they recommend, like, when you wake up or when the first thing you do at your work is you do a math problem a little bit, maybe half an hour to sharpen your mind. Your mind has to be sharpened to your brain and your mind too, you know, meditation is good. I would not allow this to make me stupid. That would be the answer.
00:34:43
I really appreciate that. And I kind of wish that everyone had that attitude, but I'm not naive enough to think that they do. Because, I mean, you're talking about having that self -regulation and the drive to become better, you know, and also being able to kind of like self -reflect and realize that if I rely on this too much, then I'm not growing and learning. so it can't grow and get better. I don't know what the solution is to train people into that curious mindset, but I definitely feel like that's something that's gonna have to happen along with this.
00:35:21
That's a problem with, I don't wanna, there's a problem with our educational system, and it's all over the world. I saw it in the best institutions in the United States, and I've also seen other parts of the world. I saw it even at Carnegie Mellon. I saw it at Georgia Tech. So I'm sure you are staying in Northeastern, right? I
00:35:44
think as you indicated too, we have to want to get better, you know, and that's maybe you can say like, you know, some people are born with that drive, but I think it is something that can be nurtured in almost anyone. I don't want to generalize, but a lot of society becomes geared around making things easier and so one of the things is to make us not have to think about certain tasks. So a little more philosophical, I wanted to ask you based on that, how do you define success for yourself personally and professionally? Do you think that there's a way that you measure it and if so, do you find that differ in your personal and professional life? Or do you think it's just kind of one guiding philosophy?
00:36:36
No, I don't know how to say this, but I think or I want to think that I've been trained in the best place in the world. I've worked for some of the best companies in the world. I haven't found what I was looking for, right? Like to side you do, right? And I think that Like, that's not like what makes you happy or what makes other people happy may not be what makes you happy, right? So in my case, how I define it is like for me, a corporate job or being because my boss, my last boss was a VP. And I was thinking, do I want to be a VP really? Do I want to be with him?
00:37:17
So I better don't ask him for advice because I don't want to be like him, right? so why to ask somebody that is not in the place where I want to be ask for an advice. My advice is follow your feelings. My intuition tells me that the only way of me being happy is really doing something transcendental. And that means at least if it's going to be a little thing, but in my philosophy or the way I see the world, the only way to transcend and really have a good life is really helping other people. Your existence doesn't have a meaning in the same way it's not in terms of the existence of other people. When I was at my job, my last job, I was saying, am I really helping these people in Boston or are we making more money? And I'm trying to say that we're helping them, right? Because the marketing would say something. And when I saw the things in the financials, I said, I don't think this is what they are saying. So that's That's
00:38:21
drives me. Like, I want to be really something meaningful.
00:38:25
That's amazing. Yeah, I think that's very well said. I mean, you know, the quote from that is, how to have a good life is helping others. And I think that's extremely well said, right? I think, you know, no matter what you're doing, I think that's a lot of what people are trying to do in their day to day. And it does lead to some amazing fulfillment. So appreciate that answer. And I think it really resonates a lot for myself, and I'm sure a lot of our community as well.
00:38:47
We did have a question from Justin. I'm going to just read it aloud real quickly. He's just curious about how long it took you to become a senior data scientist and kind of the level of effort and energy required. And I guess a little bit, I'd assume, and Justin, I don't want to put words in your mouth, but I'd assume it's, what was that path that you took as well?
00:39:06
Well, at some point I was desperate to change fields, to move fields, because I didn't want to be in consulting anymore. What I did is the theoretical or the more like straightforward approach that was doing like a master's degree, but at the same time I was doing a bootcamp, so I was doing that theoretical part and then the practical part. And that was very helpful. It took me like maybe, to be a senior data scientist, it takes time. If you want to do it well, really well, it will take around five years at least. I don't know if that answered the question. I can share with, if someone is interested, they can send me an email or send me a message on LinkedIn or whatever, and I can give you a list of resources that I would use if I were to begin from the ground up. And then that could shorten your experience because the problem is that there's so many resources and so many things that tells you that I'm going to make you a data scientist in six weeks, right? That's not true. That's not true, right? Well, at least to be a good data scientist. If you want to be a .fit predict data scientist, then yes, you can copy and paste code. Oh, it works, right? Yes, it's going to work, but is it really working? I don't know.
00:40:26
Well, I think you make a really good point too, because as we were talking about before, people want a faster, easier road to everything, but it depends on what level you want to be able to think and create and analyze, right? So I guess building off of Justin's question, because I was kind of curious about this, looking at your LinkedIn profile, how did you choose the schools that you went to? Were they your first choice?
00:40:54
Were, you know, I mean, as much or as little as you wanna share, I know that path looks a little different for us.
00:41:01
I used what I learned in, like when I was studying philosophy and that to understand knowledge, right, and there's something that's called epistemology and if you understand knowledge and how it connects then you can understand how good or you can evaluate the program how good or not. You see the other thing that I do is like I really think, for example, when I see these degrees that are a mix of analytics with an MBA, right? And it's like, they have like a subject that is like professional speaking. Okay, that's good. Like, is it really good to take professional speaking and pay $10 ,000 for these subjects? Or it's better to go to those masters that they're experts on that and better instead use that to study something that I'm not gonna be able to find in other place, right? That's just like some of the things or the criteria that I use to choose the other thing, it's of course I look for the ranking, right? So if I'm going to spend the same amount of money at the same time and if I were to choose between a university like Carnegie Mellon or I don't know, I don't want to say for other, right? You need to see what are the advantages of going. I don't know. The other thing that I think somebody would need to take into account is, is it really what you want to do? Because there's this anecdote of somebody that they went to Harvard or Carmel or things like that. Is it a good school? Yes, it's a very good school. It's one of the best in the world.
00:42:37
Is it going to make you happy? That's a different thing, right? Because it can mean a lot of suffering. So are you willing to pay that price to suffer to be able to get what you want? And And always a price. So you need also to balance that. And it depends on the place in your life where you are, right? If you have a family, if you have a child that you need to take care of. There are thousands or maybe, yes, like hundreds of posts on Reddit that you can read about, for example, the programs at Georgia Tech and where there are guys telling, hey, I'm losing my hair. I just had a hemorrhoids. You know, I'm marrying with, I'm just killing myself. I don't know if this is worthy. You need to think about it. If it's really worthy, it's very, you learn, yes, of course you learn. Is it worthy? I don't know. There are shorter paths. That's the reason why it has to be,
00:43:30
like you need to know really well what you want and from there design what you're gonna do to arrive to that place. I will not take like a silver bullet. There's no silver bullet. That would be my, but I can share resources if somebody is interested. If Justin is interested, I can share resources that I would use to shorten that path. Do you mind
00:43:54
if we share your LinkedIn or email or whatever? I mean, whatever you would like, how people could best connect.
00:43:59
Yes, the only thing that I don't share is my OnlyFans. Everything, yes.
00:44:05
That's fine.
00:44:06
We'll save that for another time. It's AI generated, so... Perfect. Just printing money, apparently, then. Yes, exactly. I will say, I think this brings up, this is certainly, we're coming towards the end of times, it's certainly a topic for another time and date, which I think would be really interesting to dive into is thinking about to your point of like skills -based versus school -based, right? I think basically what you're saying is that in some ways the school absolutely matters if that's the right path for you. However, if it's what you want to do and you're passionate about it, there are certainly other ways to get to that end goal without investing the money, going to the best school, because you're going to want to do it regardless. And so, you know, thinking through, you know, the juxtaposition between the two. And think to that point, like the underlying tenant is the same as be
00:44:56
passionate about what you want to do, know what you want to do and find out how to get there regardless of a path forward and to that point, leveraging people along the way that can help you get there quicker, because learning from other mistakes is probably the best way to learn. But as we wrap up, there's one last question that we always ask with any of these. What words of wisdom do you want to leave us as a community with today? You know, it could be a mantra. It could be a quote, advice that someone gave you, advice that you give others.
00:45:22
I think that Nancy likes very much this phrase, and I'm going to apply the same that I've said before. In this context, it's better to be approximately correct than precisely wrong. And it's the same with AI. It's better that you use and be approximately in the field, but don't use it and be like completely wrong.
00:45:42
And the same happens with mathematics and everything. Maybe I don't have the answer, but I'm near. It's better to be near than to have nothing.
00:45:51
I it's a very good life lesson. Definitely something that can be applied broadly to other aspects of life. Well, thank you so much Guillermo for taking so much time with us today and for everything that you shared with us, personal, professional, everything that you taught us. I think we all feel just a little bit wiser, maybe know a little bit more about the future of AI. If people would like to keep this conversation going with you, what is your preferred method of contact?
00:46:25
You can use LinkedIn. Later, if they reach out to me there, I can also share my WhatsApp, my whatever. I use almost everything.
00:46:34
Okay, sounds great.
00:46:35
So I'm open to reach out, please. I'm happy to help.
00:46:41
Wonderful. We appreciate that. And please let us know if there's a way we can be supportive of your journey as well. And thank you for participating in past conversations and thank you to everyone who joined and listened today. We really do appreciate you bringing your perspective and energy to these discussions. And the team behind Founders Voyage feel really fortunate to be part of this community with you and for the opportunity to bring you this cooperative learning experience each week. So if you have nominations for future interview guests or topical conversations, please feel free to suggest those. And if you'd like to support us becoming a podcast, which Spencer's been working super hard on, you can donate to our Patreon. So I'll make sure that I share Guillermo's LinkedIn profile, and then I also share our Patreon in the questions and discussion channel. And thanks again, everyone for joining.
00:47:41
Thank you guys. I really appreciate it.
00:47:43
Thank you so much. It's a pleasure. You've just finished another episode of Founders Voyage, the podcast for entrepreneurs by entrepreneurs. The team at Founders Voyage wants to thank you from the bottom of our hearts. We hope you enjoyed your time with us. And if so, please Please share this with someone else who might enjoy this podcast. You can also support us by leaving a review on Apple Podcasts and Spotify, and by donating to our Patreon. Outro music today is Something for Nothing by Reverend Peyton's Big Damn Band.